AI in Qualitative Data Analysis
Qualitative research studies the human experience by trying to answer the "why" behind people’s words and actions. Until recently, the ability to truly understand our motivations and experiences – the essence of what it means to be human – was thought to be an exclusively human endeavor.
Yet, with the growing adoption of AI in qualitative research, we face new questions about the future role of this technology in our field: Will AI become a valuable partner for qualitative researchers, or does it have the power to replace us altogether?
This article explores the current state of AI in qualitative data analysis. We'll separate myth from reality, positive from negative, and highlight how AI is already being used by researchers today.
Tl;dr - How to Think About AI in Qualitative Research
What will qualitative research look like in an AI-focused future? No one seems to agree on exactly where we are headed. However, here’s a brief overview of where we stand today:
AI tools like ChatGPT can be useful for qualitative research, but they shouldn't replace the researcher's role. Similar to how coding helps analyze data, tools like Open AI’s ChatGPT can provide initial, surface-level insights in seconds. However, your knowledge and unique human insight are critical to interpreting and making sense of that data.
AI can possibly save time compared to manual coding. Some QDA tools already boast AI-powered automatic coding for qualitative analysis, and they promise to save time, too. But the jury is still out on whether AI-automated coding actually saves time. AI can produce helpful content, but it doesn't produce publishable code or deep analysis. That is to say, reviewing AI outputs might take longer than actually doing the work upfront.
There are different ways to use AI for qualitative research. AI can be used for informal coding, summarizing large portions of data, brainstorming with your data, as a second set of eyes, or as a starting point for building a codebook. It can potentially also help formulate research questions or interview guidelines.
AI might not be ideal for all research approaches. Methods that rely on turning raw data into themes, such as any inductive analysis that builds themes from the ground up, may not be suited for AI. Despite its advancements, AI still isn’t able to “read between the lines” of human interactions (a defining feature of inductive analysis).
AI can help with inductive analysis, but not take over entirely. It’s very good at the "grunt work" of deductive coding and summarizing data, freeing up time for more interpretive analysis. Tasks formerly reserved for junior researchers may soon be handled by AI, which we address later in this article. AI can also assist with brainstorming and suggest potential themes through query-based analysis. However, the researcher still needs to interpret these suggestions in order to draw meaningful conclusions.
AI can't do the most interpretive parts of research. Will AI force (or allow) us to abdicate our role as meaning-makers? As the researcher who does the reading between the lines, you ultimately assign meaning to your data. AI can process and recognize data patterns quickly. However, it can’t interpret these patterns in ways that reflect human judgment and cultural contexts. These tasks require understanding and adaptability where AI has no reference point.
AI will challenge the traditional way of analyzing qualitative coding. It’s undeniable that AI tools will expedite tedious aspects of analyzing data, identifying patterns, and even discovering new insights. Thought of as a research assistant who takes the lead from you, AI can probably add depth to your code but not create it for you outright.
Beware of AI “hallucinations,” or blind spots. We all know about hallucinations, where AI can produce false information. However, the more insidious side of that is that AI is simply "too eager to please.” Instead of admitting it doesn’t know an answer or have enough information, it can cherry-pick facts and bend the truth to seem helpful. It’s a form of selection bias, where AI's responses are subtly skewed by your inputs. Long story short, always double-check AI outputs, especially in tasks needing a deep understanding of your data!
We’re still learning how AI can be used for in-depth analysis. AI can generate interesting results, but its usefulness depends on the project goals and the learning curve involved with the actual AI tools. The tools are still being developed to allow for helpful AI and human collaboration. And no one has cracked it yet! Developing new skills to understand and leverage AI's capabilities will be important for new researchers.
What We Mean By “AI in Qualitative Data Analysis”
Imagine a research assistant who can read mountains of qualitative data in seconds, not days or weeks. That's the power of AI in qualitative research, specifically large language models (LLMs) like ChatGPT. In this article, when we use the term "AI,” we’re mostly referring to LLMs.
Key Terms for Understanding LLMs
To grasp how LLMs work, it helps to have a layman's understanding of two basic building blocks of this tech: natural language processing (NLP) and machine learning (ML).
Natural Language Processing (NLP): This technology teaches computers to decode and interpret human language. It analyzes textual data by looking at how words are connected, how sentences are structured, and even the emotions they might convey. NLPs underpin everyday tech like Google Search, which uses them to understand our search intent.
Machine Learning (ML): Allows computers to learn from examples and improve over time. This involves analyzing data processed by NLP to find patterns and make predictions about new data without explicit instructions. However, they don't "learn" in a conscious, human-like way; they require human input to set up, guide their learning process, and make adjustments based on performance feedback. For example, an email spam filter can use example spam emails to predict if a new email is spam or not.
Large Language Models (LLMs) Combine NLP and machine learning in a novel way. An LLM is trained by having the model predict the next word in a sentence. This simple method, when trained on massive amounts of data, can produce (or create the illusion of) a form of intelligence. There is still debate if LLMs truly model actual understanding and intelligence or if they are nothing more than a stochastic parrot and “glorified auto-complete.”
What is not up for debate is that we can hold a “conversation” with LLMs like ChatGPT and Gemini from Google. They can provide timely, personalized responses that are contextually relevant and seemingly thoughtful, much like a human would. This integration allows for a more natural, user-friendly interaction with AI, bridging the gap between human and machine communication.
AI is Qualitative Research Is Not All-New
But using AI for research isn’t an entirely new concept. Researchers have been using tools built on NLP and machine learning for years to help analyze qualitative data. For example, Delve includes a project search feature that uses NLP to gather search results. This is just one of many examples of how this technology has been used by researchers to assist with coding or organizing their data in the past.
Current Tools Using Machine Learning in Qualitative Data Analysis
While ML tools have existed for quite some time, analyzing interviews and surveys with them used to be a hugely technical challenge. They often required technical expertise just to operate and lots of “training data” to run, and interpreting results was an equally technical process. LLMs have wrapped this technology in a user-friendly, conversational, query-based interface that can assist with AI research.
Qualitative data analysis (QDA) companies are also figuring out how to integrate AI into their existing products. Some have already customized GPT models to their users’ specific needs. But, these AI features are still in the early stages and still require a human to function in a coherent way.
Today, AI can’t deliver fully automated research results or fully automatic coding in qualitative data analysis. Whether it can reduce coding time will be an ongoing debate. While AI can be a valuable research assistant and a second set of eyes, it struggles to capture the "bigger picture" of how data connects.
Qualitative analysis doesn't have to be overwhelming.
Take Delve's free online course. → Get started here.
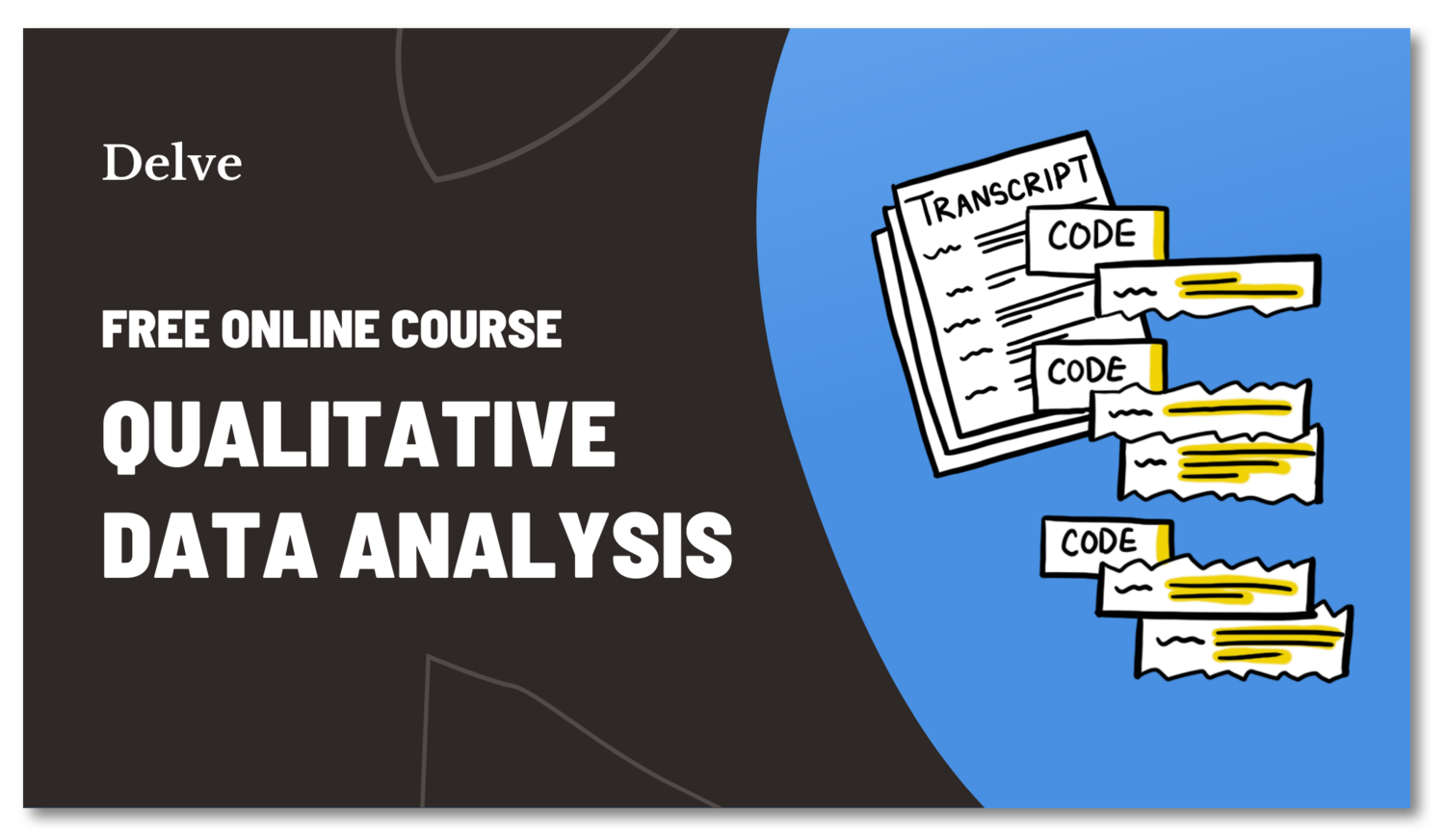
Why AI Struggles With the “Bigger Picture” of Themes
While AI can be great at data analysis, it currently struggles with thematizing data, a core aspect of qualitative research. Thematizing involves identifying underlying patterns and meanings in data, and grouping them under a central concept – the "bigger picture."
Themes are created by “patterns anchored by a shared idea, meaning or concept” (Braun & Clarke, 2021). They represent multiple analytic insights grouped under a central, organizing idea—the overarching story of the data where you connect ideas. A theme shouldn’t be mistaken for a topic summary, which is a code that just sums up everything participants say about a certain topic. Left to its own devices, AI will tend towards simple topic summaries.
For example, a topic summary like “using Zoom for remote work” really just summarizes a group of codes related to this subject. A theme should capture more nuanced insights, such as “how technology adoption affects work-life balance.” Locke et al., (2022) call this interpretative process “putting patterns together” or “progression toward theorizing.”
Methods like inductive thematic analysis, grounded theory, and reflexive thematic analysis require a deep, human grasp of pulling patterns together to build themes. And despite some QDA tools promising ready-to-publish results at the push of a button, they can only generate topic summaries, descriptive themes, or codes that barely scratch the surface of the data's true depth.
Here are some other considerations for using AI to generate themes:
Overhyped Capabilities: Instant, in-depth insights have proven to be more ambitious than achievable. Similar to topic summaries, AI-generated themes often miss what the data actually tells us about being human. These tools perform well with many tasks but they still need a human for more interpretative tasks like theme building.
Devaluing Human Research: As sides negotiated about AI script writing in Hollywood, the focus shifted from creativity and human insight (the heart of storytelling) to cost-cutting measures. In the same way, overhyping AI in qualitative research risks diminishing the value of human researchers. Meaningful analysis, like meaningful scriptwriting, hinges on the human touch in a way that interprets data beyond just processing it.
While AI helps handle and analyze data, the complex task of weaving raw data into coherent themes (that accurately represent the human experience) remains a particularly human task.
AI's Position Within Different Philosophies: Constructivism vs. Positivism
Let's explore how AI fits into two popular research philosophies, positivism and constructivism, to understand how and where it can best support qualitative researchers today.
Positivists, who believe themes are preexisting truths with or without our guidance, may be able to use AI as a powerful tool for unearthing them. It would be like using a treasure map to find buried treasure. This philosophical approach aligns with AI's ability to analyze large datasets and the deductive approach of positivism with clear hypotheses and fixed conclusions.
But in constructivism, themes aren't set in stone. We create them through our active engagement and interpretation of our data. It’s like the old saying, “The journey is the destination.” There's no pre-existing treasure map – we create meaning as we explore the data. AI just isn’t made for the personal interaction and subjective analysis of constructivism and inductive research.
In general, constructivism is more philosophically aligned with qualitative methodologies. For us to say that AI can fully replace human researchers, it would require either:
Believing that AI has enough understanding about society, the human experience, and any individual researcher’s perspective to construct meaning in the same way we would.
Rejecting constructivism in favor of the positivist perspective that there is a ground, singular truth that the AI can discover on its own, without human intervention.
Table: Framing AI Within Constructivism vs. Positivism
Aspect | Constructivism | Positivism |
---|---|---|
Approach to Learning/Researching | Active engagement to create meaning. | Analyzing clear data to draw conclusions. |
Role of the Learner/Researcher | Central, interpreting data through personal and societal views. | More detached, focusing on objective analysis. |
Data Interpretation | Influenced by individual perspectives and contexts. | Based on tangible evidence and accepted facts. |
AI's Compatibility | Struggles with subjective interpretation. | Thrives in analyzing data and identifying surface-level patterns. |
View on Knowledge Creation | Knowledge is constructed through experiences. | Knowledge is derived from objective methodologies. |
Deductive/Inductive Orientation | Inductive: Building understanding from the ground up. | Deductive: Applying general principles to specific instances. |
The Human Advantage
Qualitative research often draws from constructivism and positivism. But the constructivist approach, with its emphasis on subjective interpretation, human experience, and reflexivity, is where the human researcher brings the most value. AI can be a powerful tool for analyzing data, but it can never directly take over constructing meaning from a uniquely human perspective.
Think of AI in qualitative research as an advanced qualitative coding assistant. It’s great at organizing and sifting through large amounts of data, similar to how researchers might do rounds of initial coding. AI can quickly identify patterns and categorize information, potentially saving you time. But just like coding sets the stage for deeper analysis, AI can't replace the human perspective.
The real magic happens when we interpret AI's findings and extract the overarching themes of that data—something AI has not shown it can do. This researcher-AI partnership strengthens the research process but does not remove us from the equation altogether.
Benefits of AI Qualitative Analysis Tools
AI, specifically large language models like those used by ChatGPT and Gemini, are revolutionizing how we interact with information. They are great tools for handling the “grunt tasks” that take time away from more interpretive tasks. As of today, AI can be used to:
Explore and familiarize yourself with your data: After conducting interviews, you may have a wealth of data with emerging patterns waiting to be discovered. AI can help you explore these initial ideas and connections.
Assist with coding: AI can be a helpful tool for initial coding or deductive coding with a well-defined codebook. AI can process and recognize simple patterns in data at an impressive speed and scale compared to manual coding alone. Think quantity not quality.
Generate summaries and identify connections: Once you’ve coded your data, AI can help you explore the relationships between codes you may have missed and identify new patterns. AI can also summarize large portions of data into a type of cliff notes.
Support report writing: Having an AI writing assistant can be helpful for anyone. It can help summarize key points, help you brainstorm, clean up grammar, and help you find relevant quotes. Using AI as a dedicated editor is a great way to polish your write-up.
Disadvantages of AI Qualitative Analysis Tools
While the potential of AI in qualitative research is undeniable, these tools are more a collaborator than a direct competitor for qualitative researchers. Let’s look at some of the limitations of AI:
AI cannot create themes: AI is good at identifying simple patterns within existing data, but it cannot generate new insights or interpret the meaning behind the data. Human researchers are still required to draw insightful conclusions and themes. AI is looking to please you. It will try its best if you ask it for a theme, but what it returns will likely be a topic summary, not a theme.
AI may struggle with complex methodologies: AI might struggle with qualitative methodologies that rely heavily on a deep understanding of the data, like grounded theory. These approaches often benefit more from human intuition and interpretation.
AI needs clear communication: The quality of your inputs determines the quality of AI’s outputs. For example, think of using AI with a pre-existing codebook. Having well-defined codes and clear coding guidelines will significantly impact how well AI performs when applying that codebook. Clarity is key.
AI is missing context: Qualitative analysis isn't just interpreting the text. Research projects require a deep understanding of the research objectives, the context behind participants, the goal behind the study, and the researcher and participants lived experiences. AI doesn't have this human-level context when coding your data.
Will AI Replace Qualitative Researchers?
Ultimately, whether you think AI can replace human analysis in qualitative research hinges on your beliefs about how meaning is created and your expectations for future AI developments.
Here’s how we see things: Conducting an interview, understanding cultural nuances, and piecing together narratives will remain uniquely human tasks. These tasks don’t just require skill but the unique perspective that comes with being human. From a constructivist perspective, we are a necessary part of the meaning-making process. It’s not just about learned knowledge or even superhuman computing power but the unique insights that only we can provide.
As AI takes over the grunt work, a new frontier opens for junior researchers. The focus will shift to higher-level analysis and narrative storytelling from data. It’s a good idea to start honing your communication skills and the ability to translate data insights into clear narratives now. Communicating with other people and being able to communicate with AI is going to be key to the future of qualitative research (and future competitive advantages).
Worrying that AI could "hollow out" the research profession is understandable! If AI takes over all the junior-level work, will there be any demand for new researchers? How do new researchers gain experience? We are deeply concerned about this outcome, and view it as the most likely negative impact of AI in qualitative research. The only silver lining is that AI could make research projects more accessible for junior researchers. Allowing Ph.D. students to use AI to take on much more ambitious projects, freeing them to delve deeper into truly groundbreaking research they wouldn't have had the resources for before.
We believe that AI is a research partner and an incredibly valuable second set of eyes, but it is not a replacement. Market incentives will push to replace human researchers with inferior AI replacements. However, as a research community, we must emphasize human researchers' irreplaceable value to qualitative analysis. We can leverage AI's strengths together while safeguarding the human element that defines and protects our field.
The Future of AI and Qualitative Research
The future of AI in qualitative research is a story still being written. But one thing is clear: AI is here to stay.
At Delve, we believe in the enduring value of human-centric qualitative research. This has been our guiding ethos as we continue to develop and test our new suite of AI tools. These tools are designed to augment your research capabilities, not replace your critical judgment and creativity.
References
Locke K., Feldman M., Golden-Biddle K. (2022). Coding practices and iterativity: Beyond templates for analyzing qualitative data. Organizational Research Methods, 25(2), 265–284 https://doi.org/10.1177/1094428120948600
Braun V., Clarke V. (2022). Thematic analysis: A practical guide. SAGE
Morgan, D. L. (2023). Exploring the Use of Artificial Intelligence for Qualitative Data Analysis: The Case of ChatGPT. International Journal of Qualitative Methods, 22. https://doi.org/10.1177/16094069231211248
Cite this Article
Delve, Ho, L., & Limpaecher, A. (2024a, April 28). AI in Qualitative Data Analysis https://delvetool.com/blog/ai-in-qualitative-data-analysis