Deductive and Inductive Coding in Qualitative Research
Think of qualitative coding like exploring a new city. Deductive coding is like following a set itinerary to popular spots, while inductive coding is like combing the streets to discover new places as you explore.
When you first face your mountain of qualitative data—whether it's interview transcripts, field notes, or other materials—you have an essential choice to make. Will you analyze your data through the lens of existing theories or build your understanding from the ground up as you code all of this material?
This fundamental decision, whether to use deductive or inductive coding for your qualitative research, shapes your entire analytical journey. Your choice dictates how you interpret your data, develop meaning, and ultimately tell the story of your research.
This article walks through inductive and deductive coding. We show how to choose the right approach, how they sometimes work together, and how Delve’s computer-assisted qualitative data analysis software (CAQDAS) supports you on either path.
Feel like you're getting ahead of yourself? Take our free course to learn the fundamentals of qualitative coding – or try Delve's free trial to start coding your pile of qualitative data more effectively and efficiently.
How to Think About Deductive vs. Inductive Coding in Qualitative Research
Key Prereqs: Understanding Qualitative Coding
Qualitative coding is labeling meaningful parts of your data with tags or 'codes' to uncover patterns. The individual codes in your codebook are the foundation of your qualitative research. Codes help you trace connections across your research materials and support what you learn with specific examples. Here's how it looks in Delve:
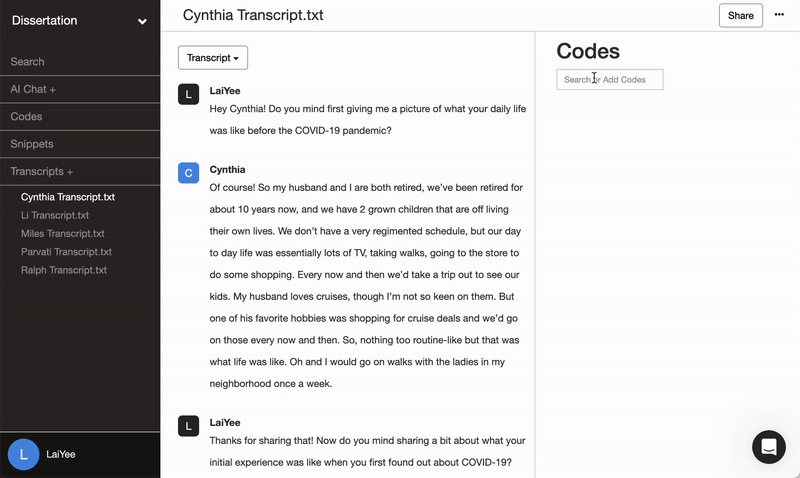
Like exploring a new city with a pre-planned walking tour versus curious wandering, there are two paths for coding your transcripts or other qualitative data. Either you’re working from the bottom-up with codes you build along the way (inductive coding) or top-down with pre-determined codes (deductive coding). Both options help make sense of your data – but in different ways:
Inductive Coding - Top Down
Inductive Coding is creating codes based on what you find in your data. Instead of starting with preset codes, you identify and code meaningful patterns through careful data analysis. This flexible approach helps you discover unexpected insights, though it requires cautious organization and tools like data triangulation to track and explain your analytical decisions.
Deductive Coding - Bottom Up
Deductive coding begins with predetermined codes based on existing theories or frameworks. You start your analysis with a good idea of what patterns you're looking for, which helps keep your work focused and systematic. This structured approach makes it easier to maintain consistency but might lead you to overlook core patterns that don't fit within your initial coding framework.
📺 Watch: Inductive and Deductive Coding in Under 3 Minutes
Choosing between inductive and deductive coding is more than choosing where to start. It's about your view on how to best uncover knowledge in your field or for your specific study. Both paths lead to understanding, but depending on your research goals, they'll take you there in different ways. Watch this quick overview (> three minutes) to learn more.
Differences in Action: Simple Example of Deductive Coding vs. Inductive Coding
Here's a simple example to show how the same chunk of text might get coded differently depending on whether you follow an inductive or deductive approach.
In this hypothetical study on stress in the workplace, Tim is a respondent who shared the following in an interview:
Tim: "I never expected to miss the office commute, but now I realize those 30 minutes were my transition time. These days, work just bleeds into everything else."
---
- Inductive Code: "Missing Transition Time" – This code arises directly from the participant's phrasing, offering a fresh perspective not found in existing research.
- Deductive Code: "Work-Home Boundary Issues" – This code aligns with established theories about work-life balance challenges and blurred boundaries.
Using Inductive vs. Deductice Analysis:
With inductive coding, "Missing Transition Time" comes directly from Tim's own words. This verbatim approach, called In vivo coding, helps uncover fresh ideas that don’t exist yet in research. While just one of many inductive coding techniques, In vivo coding helps capture meaning by using the exact language you see in your data—letting your participants' direct words guide your analysis. It might come as a surprise that Tim misses his commute with the rising remote work trend – but that’s the power of inductive analysis.
If you use deductive coding, you could use the code "Work-Home Boundary Issues" instead. This code relies on established studies and preexisting concepts about the difficulty of maintaining work-life boundaries. It places your participant's experience within a broader theoretical framework about work-life balance and occupational stress that other qualitative researchers have already developed.
Both codes tell us something important about what Tim and others went through, but they frame it differently depending on the coding approach. Whether you choose inductive coding, deductive coding, or a mixed-method approach depends on your research goals and the kind of data you're working with. The important thing is to be clear about your approach and open about it when you share your findings. Later, we’ll include a few more coding examples to reinforce these ideas.
KEY TAKEAWAYS: INDUCTIVE CODING VS. DEDUCTIVE CODING
→ Inductive Qualitative Analysis:
- Let your data guide you to fresh insights
- Track your thinking as patterns take shape
- Great for exploring uncharted territory
→ Deductive Qualitative Analysis:
- Start with tested ideas as your roadmap
- Build on what other researchers have found
- Perfect when expanding existing knowledge
Choose Your Coding Approach (Thoughtfully)
Looking at these different ways of approaching the same data shows us something important: this isn't just about how you code. Your choice between inductive and deductive analysis influences every step of your research journey, from initial reading to final themes. Starting with a clear sense of your approach helps keep your analysis focused and systematic throughout the entire process.
So, before deciding to apply an inductive or deductive lens, consider these critical details:
Research Goals and Questions: What you want to learn should guide how you code. Deductive coding helps test, extend, or refute existing theories, while inductive coding opens doors to new, unexplored theoretical insights.
Analysis Timeline: Starting with the wrong approach can mean significant backtracking. Inductive coding typically needs multiple rounds of analysis to develop robust themes, while deductive coding offers a more structured path from the start.
Reporting Requirements: Your choice also matters when writing up your results.
If you're using deductive coding, you're connecting your findings to existing theories. Results tend to sound like this: "Our data supported Smith's (2020) work-life balance framework."
With inductive coding, you build themes by listening to your data. Here, you might say: "Participants repeatedly described missing their commute as 'thinking time'—leading us to explore the role of transitions (between work and personal life)"
The important part is to be deliberate and systematic right from the start. Even if you use both methods in your research, you apply them separately and need to start with one or the other. Thinking thoughtfully about this choice avoids wasting valuable time and resources.
No matter which approach you take, tools like Delve help keep both coding approaches manageable:
Inductive Coding: Spotting new patterns in your data is exciting, but keeping track of them all can get overwhelming. Nesting codes helps you organize these discoveries into meaningful categories as your understanding grows.
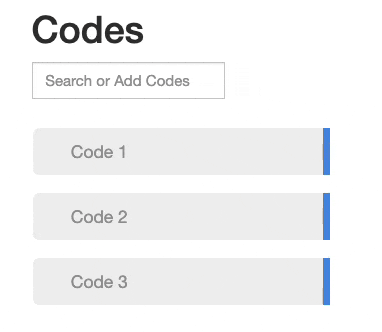
Deductive Coding: Working with predetermined codes is tricky, especially with big datasets. You can import existing codebooks and use filtering tools to pinpoint theoretical connections and locate data tied to specific frameworks more efficiently.
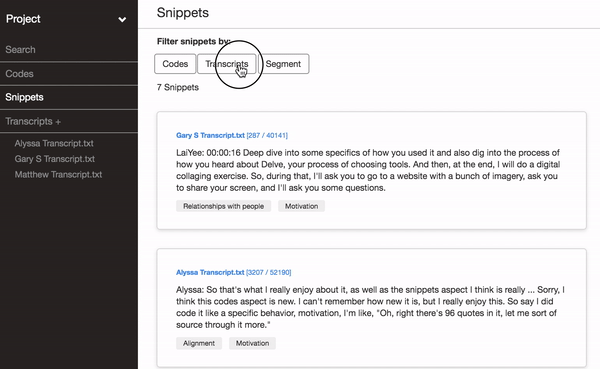
Complete Overview of Deductive vs. Inductive Coding
Now, let's dig deeper. Here’s everything you need to know about deductive and inductive coding, including when to actually use them and more information on what they look like in practice.
Deductive Coding: Starting with Theory
Top-down and focused! We’ve established that deductive coding begins with existing frameworks or theories and follows a pre-determined coding structure. While your codebook isn’t set in stone, you go into your analysis (and coding) with a good idea of what you're looking for. This approach is like a botanist with a field guide who knows exactly which plants they expect to find in the field.
Deductive coding is commonly used in methods like qualitative content analysis and program evaluation, where the goal is to apply existing concepts to real-world data or assess outcomes against pre-defined criteria. While you can also do inductive content analysis, deductive versus inductive content analysis is more common.
CAQDAS, like Delve, simplifies this process by letting you import existing codebooks and consistently apply codes across your dataset. Built-in filters and project-wide searching help ensure your analysis remains systematic, even when dealing with huge amounts of data.
Want to learn more about specific coding methods or improve your qualitative analysis? Check out Delve’s Qualitative Coding Guide for practical tips and detailed explanations.
WHEN TO USE DEDUCTIVE CODING
Consider this approach when:
You plan to test or expand existing theories
Your research questions are clearly defined by previous work
You need to compare results across multiple studies
Working within established concepts in your field
Evaluating programs against pre-defined criteria
COMMON DEDUCTIVE CODING METHODS
Structural Coding: Sorts data into categories based on research questions or themes.
Protocol Coding: Predetermined codes (based on established guidelines or protocols) are applied to data to evaluate compliance or alignment with those standards.
Provisional Coding: Begins with a “provisional” list of codes, which you adapt as needed to fit the dataset.
Evaluation Coding: Focuses on applying pre-established evaluative criteria (e.g., goals, standards, or outcomes) to assess specific elements within the data.
- Start with a clear codebook grounded in theory or existing research.
- Align codes with your research goals to maintain focus.
- Use filters or sorting tools to apply codes consistently across datasets.
- Document any changes to your codebook for transparency.
- Review your codes regularly to ensure relevance and coverage.
Inductive Coding: Letting Your Data Lead
From the bottom up! Inductive coding starts with your data and lets it show you what's interesting. Instead of coming in with preset ideas, you explore your interviews or field notes with fresh eyes. You’re staying open to unexpected patterns and stories. Qualitative research approaches like thematic analysis (inductive thematic analysis being the more common approach), interpretative phenomenal analysis, and grounded theory all rely on inductive coding because they help build new theories from real observations. Remember, you're not testing what others have found but discovering something new!
As we showed earlier, coding tools like Delve make this exploration more efficient by letting you group (or "nest") related codes together. You can quickly reorder ideas, stack codes into more meaningful categories, and carve out your final themes. This helps you organize patterns you’ve spotted while staying flexible enough to capture new insights.
WHEN TO USE INDUCTIVE CODING
This approach is particularly valuable when:
You’re exploring new phenomena or understudied topics
You want to understand lived experiences of other people
You want to stay open to unexpected insights
Your research calls for investigating understudied populations
Challenging existing assumptions or theories
COMMON INDUCTIVE CODING METHODS
Pattern Coding: Groups data into patterns for deeper analysis.
In Vivo Coding: Captures participants' exact words as codes.
Initial Coding: Lays the groundwork with broad, open codes.
Descriptive Coding: Provides a quick overview of topics.
- Start with open-ended review sessions to let key patterns surface naturally.
- Track emergent codes systematically to avoid duplication or overlap.
- Group related codes into categories early to streamline theme development.
- Use visual tools, like diagrams or tables, to map relationships between codes.
- Regularly compare new data against existing codes to refine your understanding.
Digging Deeper Into Deductive Coding vs. Inductive Coding Examples
Earlier we saw how Tim's comment about missing his commute revealed different insights through each coding lens. Now, let's look at a more detailed example to showcase how these approaches shape your analysis.
Here's what Emma, another respondent from the same workplace stress study, shared about her remote work experience:
Emma’s Deductive Coding Example
When using theories about work-life balance, start by writing clear code definitions that link directly to the theoretical framework you’re working with. Before diving in, you should create a detailed codebook that specifies when (and when not – or “negative cases”) to use each code with examples and non-examples. This information helps you maintain consistency across your analysis. If you were studying the best CAQDAS tools for students, here’s what your codes might look like in Delve:
With these definitions ready, you can begin coding Emma's interview using predetermined codes from existing studies:
“Work-Home Boundary Issues”: Emma’s struggle aligns with findings from Boundary Theory research on the challenges of maintaining clear divisions between professional and personal time in remote work environments. Recording memos help you document specific examples that fit this code and note cases where it might not apply.
“Role Conflict”: This excerpt reflects concepts from existing role conflict frameworks, such as those studied in occupational stress literature. For example, Stress Response Models identify how competing roles increase tension, making it difficult to balance responsibilities. What makes this a clear example of role conflict? Creating these decision rules in your codebook helps maintain coding consistency across your dataset.
“Coping Mechanisms”: While not explicitly stated, Emma’s experience could connect to established coping frameworks that describe how individuals adapt (or struggle to adapt) to workplace pressures. Here's where regular code reviews become crucial—test whether this code truly fits as you analyze more data, and use memos to track any adjustments to your definition.
The goal is to fit Emma’s experience into existing theoretical frameworks about work-life boundaries and transition periods. You are testing theories and then reaffirming, revising, or rebuilding what people already know. Going through this process helps connect your findings to established research but might miss some of the unique nuances in your respondents’ experiences.
Remember to periodically review your coding decisions to check you're applying these frameworks consistently! Keep a running log of any changes you make to code definitions. This transparency strengthens your analysis and helps others see your analytical process.
Quick memos in Delve help you track how theoretical frameworks fit your data. Jot down notes about perfect matches and surprising exceptions – it keeps your analysis transparent and catches those "aha" moments along the way.
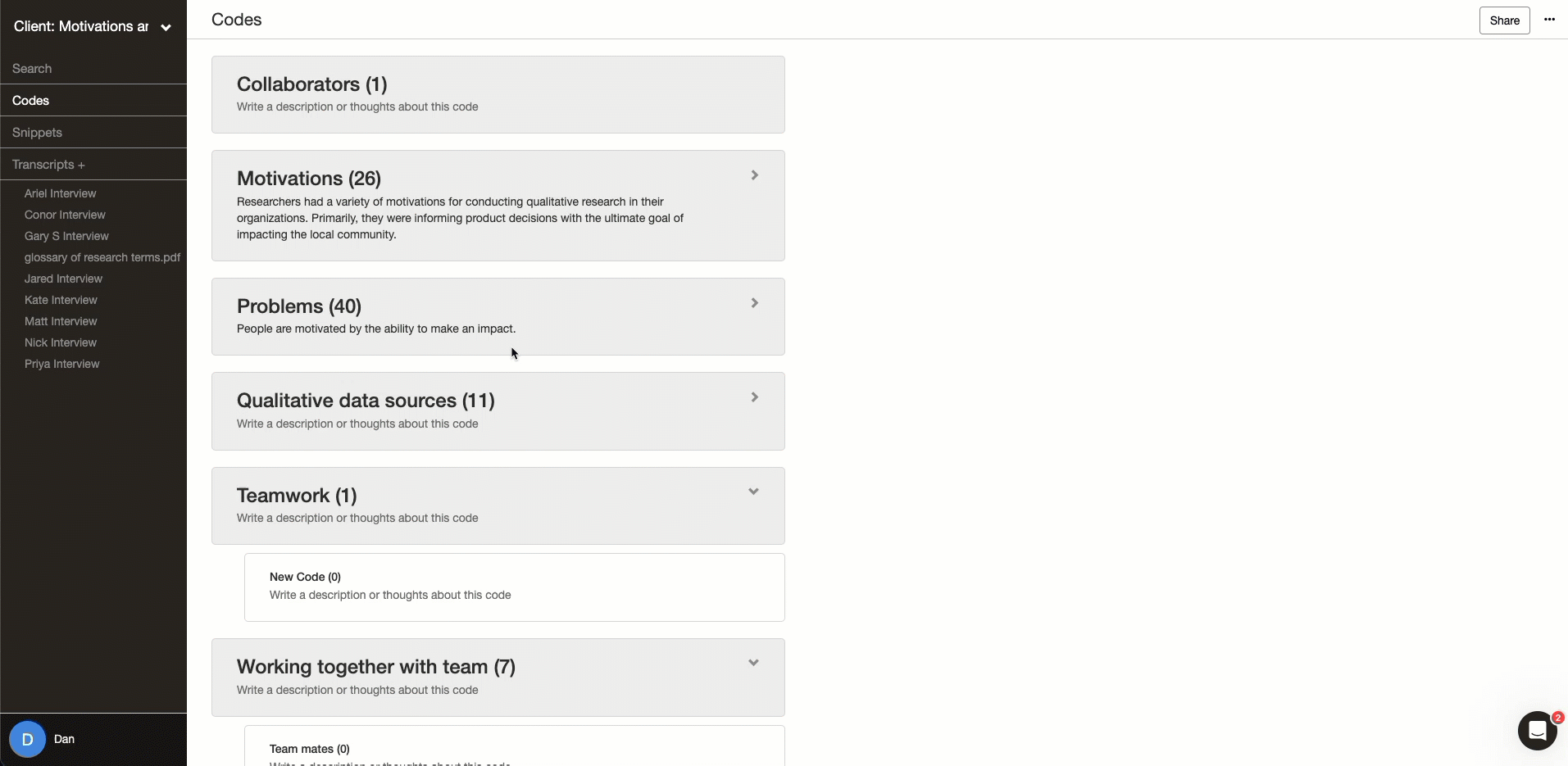
Emma’s Inductive Coding Example
When coding inductively, the process focuses on deriving insights directly from what Emma is saying rather than starting with predefined codes or ideas. You are creating new, inductive codes that reflect her unique experience in the context of stress caused by remote work:
“Endless Workday”: Captures Emma’s feeling of work extending indefinitely, emphasizing the absence of clear stopping points in remote work settings and the mental toll this creates. Writing memos about why this code was created can help track its evolution and ensure it remains grounded in Emma’s specific language and experiences.
“Struggling to Disconnect”: Highlights Emma’s difficulty in mentally stepping away from work. This code speaks to how remote work creates challenges for emotional and physical separation from professional responsibilities. Using her own words as part of an In vivo coding approach keeps this analysis participant-centered and data-reliant.
“Blurred Boundaries”: Reflects the overlap between professional and personal life, revealing the broader disruption in work-life balance caused by the shift to remote work. Keeping this code flexible early on allows it to evolve as more patterns are identified across respondents.
These inductive codes reflect Emma’s direct challenges and highlight potential patterns among other participants in the study. For instance, Emma’s experiences of “endless workday” and “blurred boundaries” build a nuanced understanding of remote work that goes beyond established theoretical models. This step-by-step process relies on comparing new data to existing codes, gradually refining your codebook as you identify patterns across interviews.
Remember, taking an inductive approach is especially valuable for exploring new phenomena like remote work challenges, where existing theories may not fully capture the nuances of people's lived experiences!
Delve makes it easy to document your emerging codes and let them grow with your analysis. Add descriptions, adjust definitions, and use drag-and-drop nesting to organize related concepts as new patterns emerge.
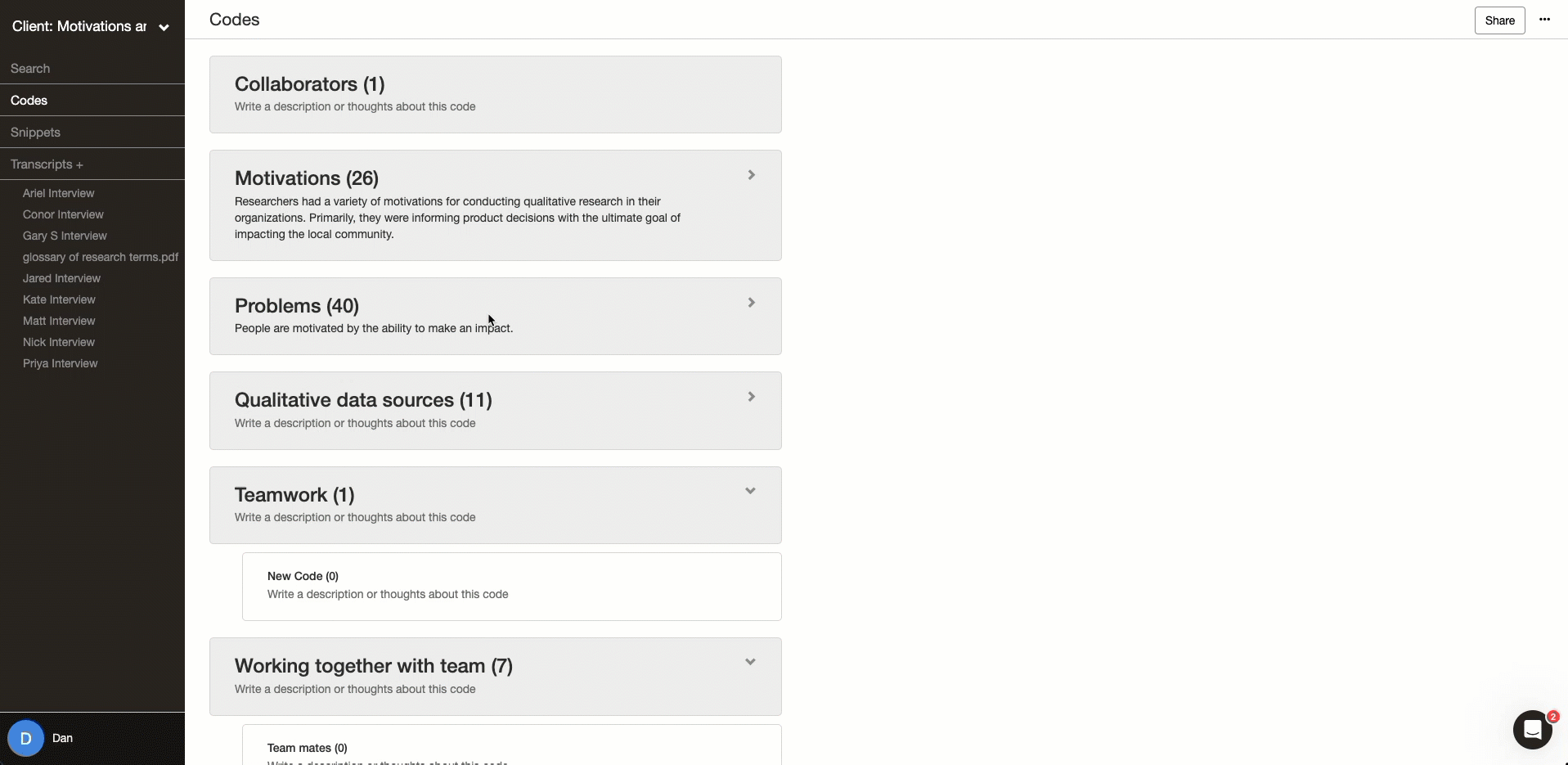
But what happens when your qualitative research requires inductive and deductive analysis? In some studies, blending inductive and deductive methods can provide a richer, more comprehensive analysis. Let’s look at how mixed methods coding can bridge these approaches to balance flexibility and structure in your research.
When to Mix Inductive and Deductive Coding
Some researchers find value in using both methods. Mixing approaches requires careful planning and clear justification. Here are the main scenarios where combining them can make sense:
Exploratory to Systematic Analysis: Start with a small sample or preliminary data to identify key themes through inductive coding, then apply these insights as a structured framework (deductive) to analyze a larger dataset. This approach is especially valuable in mixed-methods research, where qualitative exploration guides quantitative validation.
Longitudinal or Observational Studies: When following participants over time, begin with inductive coding to deeply understand their experiences. Later, use deductive coding to track specific themes across time points, ensuring consistent comparison. This approach provides a way to observe shifts and validate patterns over time.
If you choose to mix approaches, be prepared to document the rationale and methodology carefully. Track your process with memos and allow extra time, as mixed approaches often require thorough explanation and careful alignment with your study’s goals.
Common Challenges in Inductive and Deductive Coding (and How Delve Fixes Them)
Each coding approach has advantages and distinct challenges. Modern qualitative analysis software like Delve helps you overcome these hurdles, making your analysis more systematic, efficient, and rigorous. Let’s walk through some of these pitfalls – and how Delve helps avoid them.
Challenges with Deductive Coding
Deductive coding relies on existing theories and frameworks, which can sometimes limit flexibility and create obstacles in analysis. Here’s a closer look at common hurdles, along with how Delve helps researchers tackle them seamlessly:
Fitting Data Into Predefined Categories: Over-reliance on theoretical frameworks can force data into rigid categories, risking the loss of subtle nuances or other possible understanding. Delve’s customizable code descriptions ensure each category is clearly defined, allowing you to revisit and refine codes as needed without compromising theoretical consistency.
Balancing Theory With Flexibility: Staying true to your framework while remaining open to unexpected findings requires careful judgment. Delve’s co-occurrence matrices help you visually explore intersections between codes, making it easier to spot patterns that frameworks like deductive content analysis might not initially cover while staying locked into your theory.
Tracking Overlap & Complex Intersections: Overlapping or co-occurring codes can create confusion, leading to potential misinterpretation or oversimplification. Delve offers intuitive tools to organize your coding process, from nested categories to robust filtering options, ensuring clarity even when codes overlap.
Ready to dive deeper into qualitative coding?
Explore our free course and learn step-by-step how to analyze your data with confidence.
Challenges with Inductive Coding
Inductive coding provides flexibility to uncover new patterns but often requires extensive organization and ongoing refinement. Here are some typical challenges and how Delve keeps your analysis structured and insightful:
Code Overload: Without a clear strategy, you can create too many disconnected codes. This overload makes it hard to draw meaningful conclusions. Delve’s drag-and-drop nesting tools allow you to group related codes as patterns emerge, keeping your workspace clean and your codes connected to broader themes.
Maintaining Consistency: Building codes from scratch can lead to inconsistencies, especially when analyzing a large dataset. With Delve, you can write detailed memos for each code, documenting why and how it was created, which helps maintain consistency throughout your project.
Staying Focused on Research Goals: Exploring new ideas can sometimes distract you from your research objectives. Delve’s visualized coding structures make it easier to step back and connect granular insights to your overarching questions, ensuring a balance between exploration and focus.
Moving Forward with Your Qualitative Coding →
Whether choosing an inductive, deductive, or combined approach, success lies in the following:
Clearly documenting your process
Regularly reviewing and refining code
Recording memos to track your ideas
Considering peer reviewing
Staying true to your research goals
Maintaining analytical rigor and focus
Modern CAQDAS like Delve can help manage these processes and keep you on track from the start. It keeps your coding organized and accessible without sacrificing the flexibility to adapt as your analysis evolves.
Ready to start your coding journey? Get started with Delve's free trial and experience how the right tools can transform your qualitative analysis process.
References:
Saldana, Johnny (2009). The Coding Manual for Qualitative Researchers. Thousand Oaks, California: Sage.
Bath, R., Bucholz, T., Buros, A. F., Singh, D., Smith, K. E., Veltri, C. A., & Grundmann, O. (2020). Self-reported Health Diagnoses and Demographic Correlates With Kratom Use: Results From an Online Survey. Journal of addiction medicine, 14(3), 244–252. https://doi.org/10.1097/ADM.0000000000000570
Maseda, E., Ramírez, S., Picatto, P., Peláez-Peláez, E., García-Bernedo, C., Ojeda-Betancur, N., Aguilar, G., Forés, B., Solera-Marín, J., Aliaño-Piña, M., Tamayo, E., Ramasco, F., García-Álvarez, R., González-Lisorge, A., Giménez, M. J., Suárez-de-la-Rica, A., & HELP Investigators and the Perioperative Infection Research Group (2019). Critically ill patients with community-onset intraabdominal infections: Influence of healthcare exposure on resistance rates and mortality. PloS one, 14(9), e0223092. https://doi.org/10.1371/journal.pone.0223092
Florio, G. A., Donnelly, J. P., & Zevon, M. A. (1998). The structure of work-related stress and coping among oncology nurses in high-stress medical settings: a transactional analysis. Journal of occupational health psychology, 3(3), 227–242.
Cite this blog post:
Delve, Ho, L., & Limpaecher, A. (2024a, November 21). Deductive and Inductive Coding in Qualitative Research https://delvetool.com/blog/deductiveinductive