Intercoder Reliability in Qualitative Research
Intercoder reliability is like a master recipe for team-based qualitative research. Without this system, your team might end up coding like chefs cooking separate versions of the same dish. Intercoder reliability is core to a consistent and dependable team effort.
When you're staring at mountains of qualitative data - from interviews to news articles - finding the bigger picture story can be overwhelming. Even veteran researchers struggle to find their footing. But working with a team adds another wrinkle: you need to know (and show) everyone’s code is aligned.
This article introduces intercoder reliability for consistent coding – why you need it, which type to choose, and how to do it. You’ll also learn how coding software like Delve automates the heavy lifting, turning hours of number-crunching into instant intercoder results in a few clicks.
How research teams mold raw data into reliable results
The first step before measuring intercoder reliability is collecting and coding your interviews or other qualitative data: identifying and labeling meaningful patterns in your research documents
Whether you’re doing solo research or collaborative qualitative analysis, coding is a core part of the understanding process. Each code in your codebook represents a high-level idea or larger theme, like a map for your data. Conjoining these insights turns all that unstructured data into a cohesive narrative.
But when you're working with a team, multiple coders are interpreting that map. You need a way to triangulate everyone's interpretations. Using an intercoder reliability test balances out the subtleties in how you each grasp and apply your codebook, measuring how consistently you apply the code across the entire dataset.
As one type of researcher triangulation, high intercoder agreement scores establish trust. As you’ll see in the next sections, not just for your readers but for your team. These tests show that the patterns you’re finding reflect what's actually in your data – not just loosely held individual interpretations.
Modern coding software like Delve makes this process smoother, helping research teams work together effectively while maintaining analytical rigor. But before we dive into the best tools for the job, let's understand what we're trying to achieve.
🤝 Researcher triangulation: More eyes, stronger findings
Combining insights from multiple researchers leads to deeper, more reliable analysis—but managing different perspectives can get messy. Delve keeps everything organized with side-by-side coding views, clear attribution, and seamless collaboration tools. Compare interpretations, refine themes, and ensure consistency—all in one place.
What is intercoder reliability in qualitative analysis?
If three researchers apply the same codebook to the same data and consistently agree on codes, it signals a stable and believable analysis. Without that level of agreement, it’s fair to wonder: Are these insights truly grounded in the data—or just shaped by this one person’s perspective? Intercoder reliability helps you answer these questions, like a quality check for fellow coders.
Richards (2009) explains that intercoder agreement shows that you (yourself) are “reliably interpreting a code in the same way across time, or that you can rely on your colleagues to use it in the same way.” Testing and tracking how consistently you code is a trust-building activity.
When you establish strong inter-coder reliability, you're showing:
Your coding system is clear and transferable (more on this below)
Different researchers can consistently apply your codes
Your findings are grounded in systematic analysis
Your research process is transparent and trustworthy
The goal is to show others they could replicate your study’s process and confirm your results. Publishing your methods and reporting intercoder reliability scores adds this level of transparency. That is to say that consistent intercoder agreement makes people more likely to trust your results.
🔁 Quick tip: Intercoder reliability isn't about being "right"—it's about being consistent. The goal is to show that your coding system is reliable enough that different researchers would reach similar conclusions.
Quick intercoder reliability example
Qualitative content analysis tends to involve larger datasets than other qualitative methods, which makes it a prime candidate for intercoder testing.
Imagine analyzing hundreds of news articles, social media posts, or policy documents. Content analysis often requires large sample sizes to be credible and trustworthy, making consistency between coders very important when making hundreds of interpretive decisions at scale.
This is particularly important in content analysis and similar approaches where you're interpreting text, interviews, or other qualitative data. Your goal is to show that your findings are:
Credible: Based on systematic analysis rather than casual observations
Dependable: Following clear, documented procedures others can understand
Transferable: Other researchers can apply your approach in similar contexts
Confirmable: Your findings are grounded in data, not personal biases
For example, say you're analyzing media coverage of climate change across dozens of different news outlets and hundreds of articles. The goal would be to consistently identify:
Direct mentions vs. implicit references (e.g., "global warming" vs. "changing weather patterns")
Positive vs. negative framing (e.g., "innovative climate solutions" vs. "costly environmental regulations")
Scientific vs. political angles (e.g., "researchers measure ice melt" vs. "lawmakers debate carbon tax")
With thousands of these judgment calls across large datasets, even small inconsistencies between coders can significantly skew your results. That's why teams often calculate intercoder reliability on a sample first, a process that tools like Delve automate so you can check consistency throughout your analysis.
💡 Best practice: Test your coding system on a manageable sample first to ensure consistency before scaling up to your full dataset. Need a streamlined way to check intercoder reliability? Delve makes it easy—compare coder agreement at a glance with built-in reliability tools.
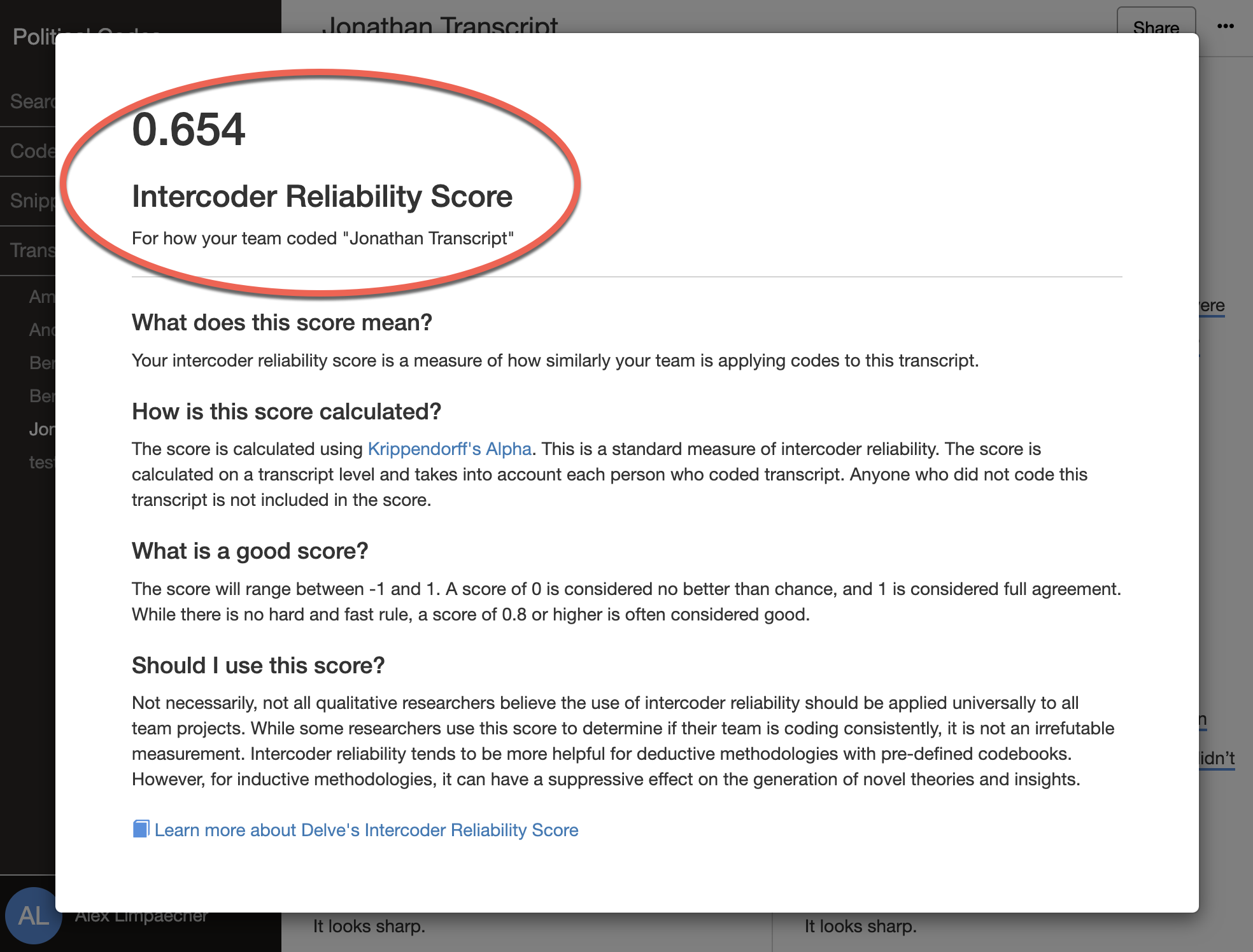
What makes qualitative research reliable – or unreliable?
Qualitative research often faces skepticism about its rigor. Critics, maybe even friends or colleagues, might ask: "Isn't this really just your interpretation?"
Reliability in research is being able to show how and why you made decisions, removing doubt, and establishing transparency in your process.
By measuring intercoder agreement, your findings aren't just subjective impressions but are based on consistent, reproducible analysis. These characteristics balance the subjectivity of the coding process from one teammate to another, letting others see that.
Think of reliability (in research) this way:
Your data tells a story about your research questions
That story needs to be trustworthy
Other researchers should be able to add your analytical path
Measuring reliability provides evidence your system works
Reliability isn’t about numbers but trust. You want others to trust that your research isn’t just a one-off, unreliable interpretation. By calculating intercoder reliability (or other forms of researcher triangulation like consensus coding or split coding), you’re essentially saying, “If you follow the same coding playbook, you’ll probably reach the same conclusions.”
Reliability builds trust in your process
In qualitative analysis, establishing trustworthiness is how you get others to believe in your research process - and there are several ways to do this. One powerful approach is triangulation, where you strengthen your findings by looking at your data from multiple angles. This can involve using different:
Data sources (like combining interviews with observations)
Methods (mixing qualitative and quantitative approaches)
Theoretical perspectives (viewing data through various theoretical lenses)
Researchers (having multiple people analyze the same data)
Strong reliability also empowers your team to tackle large datasets confidently and efficiently. With everyone coding through the same analytical lens, it’s like speaking the same language before diving into different chapters of your data’s story. You can then split up your coding workload, having confidence that everyone is seeing the data similarly and coding it consistently.
📌 Different approaches to team coding
Intercoder reliability checks consistency when coders work together. For exploratory work, consensus coding lets you code together in real time. Split coding, a type of intercoder test, starts with independent coding, then resolves differences through discussion. Learn about Consensus vs. Split Coding →
Intercoder Reliability in Qualitative Content Analysis
As mentioned in our earlier example, qualitative content analysis presents some unique reliability challenges. Even with inductive content analysis, where you have more license to create codes in your own way, you're not just categorizing huge amounts of data in a vacuum. The hurdle with collaborative content analysis is interpreting subtle meanings in text, tone, and context as a collective coding unit.
While all qualitative analysis involves interpretation, content analysis really benefits from inter coder reliability because you tend to work with huge amounts of text where subtle interpretive differences can vastly impact your results. Measuring intercoder agreement is like your team’s measuring stick, and avoids having to backtrack or recode data due to misaligned or misunderstood coding standards.
But beyond content analysis, let’s review other scenarios where researchers use intercoder reliability.
📌 Intercoder agreement made easy
Intercoder reliability calculations used to be a headache. Without the right tools, they still are. Delve CAQDAS (Computer-Assisted Qualitative Data Analysis Software) makes it simple. Teams code independently, compare results, and get an automatic reliability score—no manual calculations, just deeper analysis.
Beyond content analysis: When to use intercoder reliability?
Not every qualitative project needs intercoder reliability testing. Use it when your study requires multiple researchers to interpret data consistently and demonstrate coding reliability.
When reliability is a goal, Krippendorf (2004) suggests having at least three team members: one to design the codebook and at least two others to code sample data. This helps reduce potential bias from the person who created the coding system.
Here's when intercoder reliability makes sense – and when it doesn't:
Use intercoder reliability when:
You need multiple researchers to interpret data consistently
Your study requires standardized coding
Your publication or funding requires reliability measures
You're conducting content analysis
Skip reliability testing when:
You're doing exploratory research
You want to embrace different interpretations
You're conducting an initial discovery phase
You're looking for unexpected patterns
Inter-coder reliability also lets you divide and conquer large data sets more confidently. Once you know that your team is able to code relatively consistently, you can split the work. Each researcher takes a different portion of the data, and everyone knows that they’ll code it in a consistent manner.
Just be sure to document your process with thick description. Everyone should use memos to explain key coding decisions and how they handled tricky passages. This combination of reliability measures and rich documentation strengthens the credibility of your findings.
For more exploratory studies, like if you’re doing grounded theory, consider consensus coding or split coding instead. These approaches better suit discovery-oriented research.
📚 Beyond numbers: Thick description and reliability
Don't just report your reliability scores—document your coding journey with thick description. Show examples of how your team applied codes, explain challenging coding decisions, and describe how you handled edge and outlier cases. Delve lets you capture these insights in memos as you code, building a detailed record of your analytical process.
How to calculate intercoder reliability: A practical guide
Here's where many researchers get stuck. Traditional reliability calculations involve complex formulas, spreadsheets, and hours of manual work. Modern tools like Delve have transformed this into a more seamless and automatic process that doesn’t require number-crunching, but let's understand your options.
Choosing your measurement method
Let's walk through your options, from simplest to most sophisticated:
Percent Agreement
Best for: Quick preliminary checks and simple projects
Think of this as your starter intercoder test - simple but with important limitations.
Benefits:
Takes minutes to calculate
Easy to explain to stakeholders
Perfect for initial team alignment
Great for training new coders
Limitations:
Doesn't account for chance agreement
Can't identify specific disagreements
May overestimate reliability
Too simple for publication-quality research
📐 Formula: Percent agreement
(Number of agreements ÷ Total units of analysis) × 100
Holsti’s method
Holsti’s method measures agreement between coders when they are not coding the exact same sections of data. If coders analyze the same sections, it functions similarly to percent agreement. However, like percent agreement, it does not account for agreement occurring by chance.
Benefits:
Efficient and easy to calculate.
Works even when coders analyze different sections of data.
Limitations:
Assumes coders work independently, which may not always be the case.
Does not correct for chance agreement, which can overestimate reliability.
Not considered strong enough to be the sole measure of reliability.
📐 Formula: Holsti’s method
Definitions:
- a = Agreements on items with category presence
- b = Agreements on items with category absence
- c = Disagreements on items with category presence
- d = Disagreements on items with category absence
% Agreement = ((a + b) ÷ (a + b + c + d)) × 100
Cohen's kappa
Best for: Projects with exactly two coders
Think of this as percent agreement's more sophisticated cousin - it accounts for chance agreement.
Benefits:
Accounts for chance agreement
More credible for publication
Provides clearer picture of actual agreement
Well-established in research
Limitations:
Only works with two coders
Can be overly conservative
Assumes coder independence
May underestimate reliability in some cases
📐 Formula: Cohen’s Kappa (κ)
Definitions:
- p_o = Proportion of observed agreements
- p_e = Proportion of expected agreements by chance
κ = (p_o - p_e) ÷ (1 - p_e)
Krippendorff's Alpha
Best for: Complex research projects and publication
The gold standard for content analysis. While traditionally challenging to calculate manually, Delve’s qualitative coding tool has made this sophisticated measure accessible to everyone.
Benefits:
Works with any number of coders
Handles missing data gracefully
Adjusts for different measurement levels
Perfect for publication-quality research
Limitations:
Complex to calculate manually
Requires more methodology explanation
Higher learning curve for interpretation
📐 Formula: Krippendorff’s alpha (α)
Definitions:
- O = Observed disagreements
- E = Expected disagreements
α = 1 - (O ÷ E)
Calculate intercoder reliability made easy–with Delve
Manual calculations are not only prone to error but create severe bottlenecks in your team’s workflow. Here's how straightforward the process can be with Delve:
1. Create your project with a codebook Start with clear code definitions - your team's shared vocabulary. You can drag and drop your codebook with just a few clicks.
2. Invite your team to the project Use the 'Share' button to bring everyone into the same workspace.
3. Code independently using "Coded By Me" Everyone codes the same transcript without seeing others' work.
4. Confirm your work is complete When ready, click inter Coder Reliability.
5. Get your reliability score Get your Krippendorff's alpha score in one click with Delve!
Not convinced? Try Delve free for 14 days! [Start Your Free Trial →]
💡 Best Practice: Calculate reliability scores throughout your project, not just at the end. Regular checks help catch and correct coding drift before it becomes a larger problem that steals more time.
Wrapping up intercoder reliability
Beyond the numbers, intercoder reliability is about trust. When you can show that multiple researchers consistently agree on how to code your data, you're building trust in your findings. Not only does this rake in trust from others, but it also keeps you and your team on track to meet your deadline.
What once took hours of manual calculations is now an automated process with modern qualitative analysis software like Delve, strengthening research rigor without the huge administrative burden.
Ready to make your qualitative research more reliable? Start your free trial of Delve and see how easy measuring reliability can be.
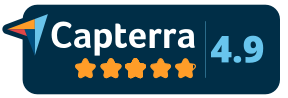
Simplify Your Thematic Analysis with Delve | Top-Rated by Researchers ⇒
Rated as a top qualitative coding software on Capterra, Delve simplifies the entire process, making it easier to uncover patterns, collaborate, and produce insights that matter.
Get 14 Days FreeNo commitment. Cancel anytime.
References:
Lombard, Matthew & Snyder-Duch, Jennifer & Bracken, Cheryl. (2005). Practical Resources for Assessing and Reporting Intercoder Reliability in Content Analysis Research Projects.
Richards, Lyn (2009). Handling Qualitative Data: A Practical Guide, 2nd edn. London: Sage.
Shenton, A. K. (2004). Strategies for Ensuring Trustworthiness in Qualitative Research Projects. Education for Information, 22, 63-75. https://doi.org/10.3233/EFI-2004-22201
Potter, W. James and Deborah Levine-Donnerstein. “Rethinking validity and reliability in content analysis.” Journal of Applied Communication Research 27 (1999): 258-284.
Klaus Krippendorff, Reliability in Content Analysis: Some Common Misconceptions and Recommendations, Human Communication Research, Volume 30, Issue 3, July 2004, Pages 411–433, https://doi.org/10.1111/j.1468-2958.2004.tb00738.x
Cite this article:
Delve, Ho, L., & Limpaecher, A. (2023c, April 26). Inter-coder reliability https://delvetool.com/blog/intercoder