What Is Consensus Coding and Split Coding in Qualitative Research?
Split coding and consensus coding are used by research teams to enhance the trustworthiness of their qualitative data analysis. These optional, collaborative coding methods are applied after an initial codebook is generated, helping to generate a “final edition” of the codebook.
The goal of both methods is to offer a transparent audit trail to explain coding decisions. You can think of them as processes of quality assurance. They help balance the inherent subjectivity of qualitative coding by reducing researcher variability in how codes are applied or perceived.
Ultimately, both split coding and consensus coding offer rigorous, systematic, and defensible coding definitions that can be implemented with fidelity on a consistent basis. [1]
Tl;dr - Consensus Coding vs. Split Coding
Hemphill (2018) states that split coding and consensus coding are both forms of “final coding.” Researchers use them at the final stage of coding to discuss coding decisions and finalize the codebook. The two coding methods differ mainly in their overall efficiency and level of rigor.
Consensus coding is when researchers code the same transcripts and compare results on a one-to-one basis. This method is more rigorous but also more time-consuming.
Split coding is when researchers divide their transcripts and code them separately. Each transcript is discussed but with less attention given to each one, shortening the process.
In both cases, researchers record notes or memos in a shared research journal. The journal tracks anything from coding inconsistencies to new insights.
Weekly meetings are held to iteratively compare new data to previously coded data to improve the rigor of analysis—similar to the constant comparative method in grounded theory.
New to qualitative analysis? Here’s a simple, step-by-step introduction.
Why Researchers Use These Coding Methods
Beyond improving trustworthiness and credibility in your study, both of these methods are particularly helpful when researchers want:
To ensure accuracy in interpreting complex datasets.
To offer unbiased analysis of sensitive or controversial topics.
To incorporate perspectives of coders from multiple disciplines.
To represent all of the researchers' perspectives within the final codes.
To reinforce intercoder reliability and ensure consistent coding of data with multiple researchers i.e. reduce researcher variability and improve the validity of your study.
[Related readings: Find more easy-to-grasp definitions of other qualitative coding methods.]
What is Split Coding?
Split coding is when researchers divide transcripts among multiple coders. Coders analyze 2-3 transcripts independently in each iteration and record notes in the research journal. In weekly meetings, they cross-check the coding scheme, review each transcript, and discuss the journal.
Split coding is more efficient than consensus coding as it allows researchers to cover more ground by coding different transcripts, albeit with less rigor in the final results. Smaller teams with limited time or resources usually prefer split coding.
Remember that what you gain in efficiency you may sacrifice in rigor, including intercoder reliability. In short, coder variability is easier to manage with consensus coding because each transcript is coded by each researcher. Transcripts are also then compared on a one-to-one basis.
For this reason, split coding relies more on the clarity of preliminary coding phases and pre-defined coding conventions than consensus coding (Gibbert et al., 2008).
When to use split coding?
As an efficient process of codebook quality assurance
To minimize issues of researcher variability
For final coding with 2-3 other researchers
How to do split coding
Summarizing the above, here are the steps for split coding:
Each researcher codes different transcripts with the pre-defined codebook.
The coders then meet to review each coded excerpt in the codebook. Using the research journal, they flesh out any discrepancies, disagreements, or differences that surface.
The team eventually comes to a consensus threshold and finalizes the codebook.
What is Consensus Coding?
Consensus coding is when researchers each code the same transcripts. In each weekly iteration of coding, the researchers code 2-3 transcripts and record their notes in the research journal. Then, they meet to compare transcripts on one a one-to-one basis and review the journal.
This process repeats itself in each consecutive round of coding until the complete dataset is checked. This is an exhaustive, time-consuming process. You can save time by using consensus coding in the first iterations and then finishing with split coding, as we will explain below.
Olson et al. (2016) add that consensus coding “is likely the more effective approach when working in larger groups where coding consistency concerns are more abundant.”
When to use consensus coding?
Consensus coding is particularly useful when researchers want:
A more rigorous process of codebook quality assurance
To pay particularly close attention to coding consistency
For final coding with larger groups of researchers
How to do consensus coding
Now that you understand what consensus coding is, here is how to apply it in your study:
Each researcher codes the same transcripts with the pre-defined codebook.
They review each transcript and compare results on a one-to-one basis in the weekly meeting.
They also review the research journal to flesh out any discrepancies, disagreements, or differences that surface before moving on to the next iteration.
They repeat the process until all the data is coded and finalize the codebook.
Qualitative analysis doesn't have to be overwhelming
Take Delve's free online course to learn how to find themes and patterns in your qualitative data. Get started here.
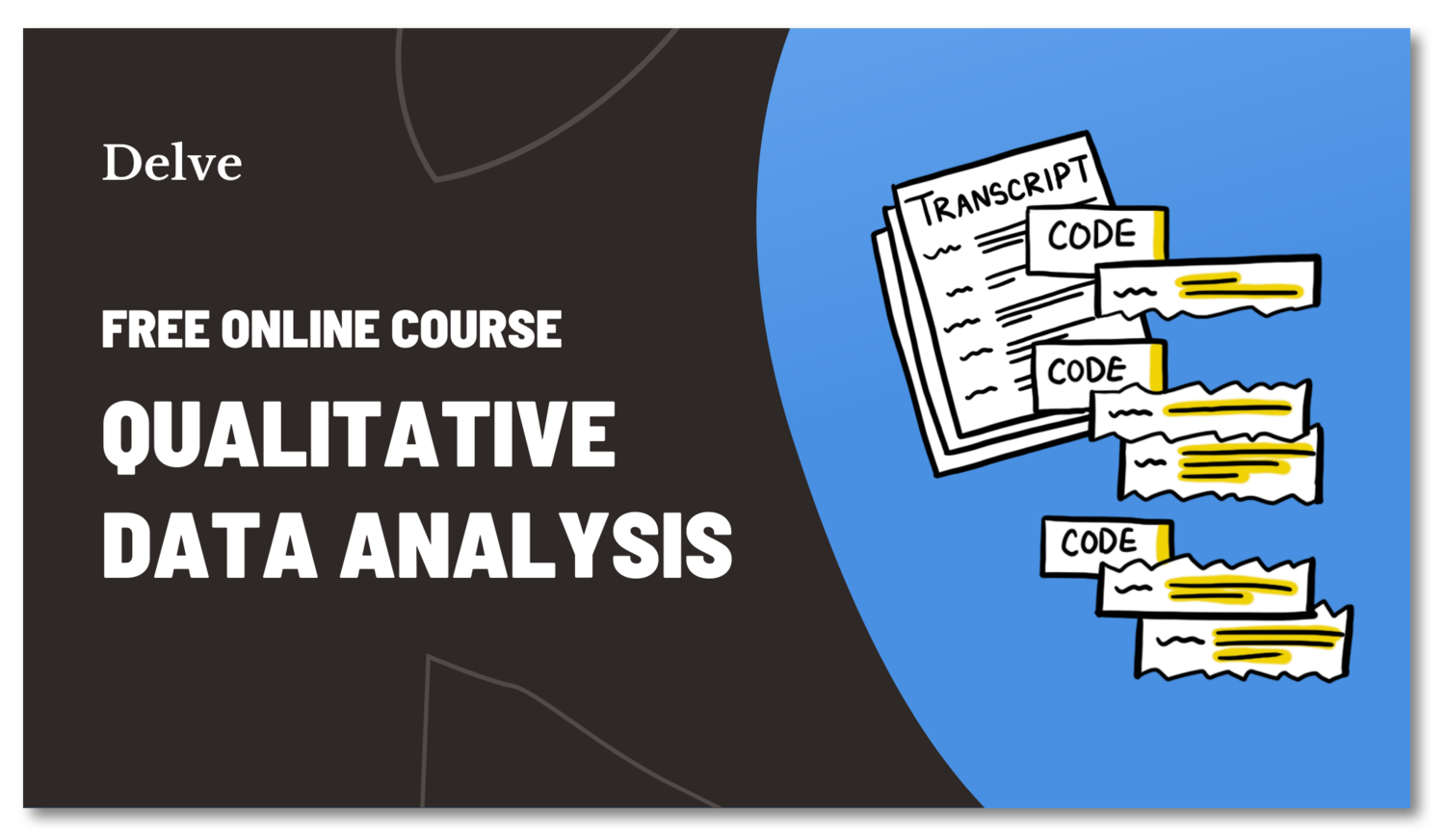
Combining Consensus Coding and Split coding
The main reason to combine these methods is to apply the rigor of consensus coding in the first rounds of coding and the efficiency of split coding in later rounds.
You still get feedback from all of the researchers and utilize the best of both methods. Basically, once you have found enough of a consensus, you don’t need everyone coding every transcript.
Here is how a hybrid approach to final coding works:
Use consensus coding to find alignment on coder variability.
Once you reach a consensus threshold, use split coding to code the rest of the data.*
Continue with the weekly meetings until completion and finalize the codebook.
* It is important that each researcher voices their opinions or concerns before shifting to split coding. If there is still uncertainty or disagreement, you may jeopardize the intercoder reliability of your study. You can use the rounds of consensus coding to ensure coder consistency.
A Note on Coder Disagreement
Beyond coder variability, researchers may not always reach a full consensus or agreement on each coding decision. Luckily, these coder disagreements are often constructive. In fact, many researchers argue that, while time-consuming, inter-coder disagreement adds rigor to a study.
The main idea is that disagreement elicits discussion and debate. Zade et al. (2019) note that while it may be challenging to explore and build understanding around disagreements, the process often yields unanticipated insights from the data. In this way, disagreement is predictable, correctable, and contains hidden but helpful information. [5, 6]
Lastly, split coding and consensus coding are also valuable learning devices for novice researchers who are still new to the process of qualitative coding.
Beyond Triangulation: Similarities Between Split Coding and Consensus Coding
You can think of split coding and consensus coding as different types of researcher triangulation where multiple researchers work together to collect and analyze data. Beyond this similarity, here are a couple more benefits of using these optional coding methods:
Both coding methods use group discussions as an open forum to provide a deeper, more meticulous understanding of the data.
Both can be applied to various sources of data—such as interviews, focus groups, text, and observations—to identify patterns and themes.
Both can enhance the transparency and transferability of your results.
To address transferability, you present a detailed account of the codebook in the final write-up (Shenton, 2004). This account makes it easier for other researchers to vet your research process and to transfer the codebook to their own research.
Wrapping Up
Split coding and consensus coding are two optional methods of final coding. They encourage independent coding by different researchers, which can help to ensure that the codebook is not biased by the perspectives of a single coder.
Collaboration is a central facet of both coding methods. Weekly discussion help researchers discuss their findings, and find a consensus threshold on areas of coder disagreement.
In summary, researchers use these collaboration methods to enhance the trustworthiness and validity of their study and also promote learning and exchange of ideas among researchers.
Qualitative Content Analysis With Delve
Overall, Delve offers numerous benefits for researchers. From increased efficiency and accuracy to improved collaboration and customizability, the software can help researchers streamline their work and achieve more robust and meaningful analysis results.
References
Hemphill, M. A. & Richards, K. A. R. (2018). A practical guide to collaborative qualitative data analysis. Journal of Teaching in Physical Education, 37(2), 225–231. https://doi.org/10.1123/jtpe.2017-0084
Gibbert, M., Ruigrok, W., & Wicki, B. (2008). What passes as a rigorous case study? Strategic Management Journal, 29, 1465–1474. doi: 10.1002/smj.722
Olson, J.D., McAllister, C., Grinnell, L.D., Walters, K.G., & Appunn, F. (2016). Applying constant comparative method with multiple investigators and inter-coder reliability. The Qualitative Report, 21(1), 26–42.
Himanshu Zade, Margaret Drouhard, Bonnie Chinh, Lu Gan, and Cecilia Aragon. 2018. Conceptualizing Disagreement in Qualitative Coding. In Proceedings of the 2018 CHI Conference on Human Factors in Computing Systems. ACM, 159.
Klaus Krippendorff. 2008. Systematic and random disagreement and the reliability of nominal data. Communication Methods and Measures 2, 4 (2008), 323–338.
Himanshu Zade, Bonnie Chinh, Abbas Ganji, and Cecilia Aragon. 2019. Ways of Qualitative Coding: A Case Study of Four Strategies for Resolving Disagreements. In Extended Abstracts of the 2019 CHI Conference on Human Factors in Computing Systems (CHI EA '19). Association for Computing Machinery, New York, NY, USA, Paper LBW0241, 1–6. https://doi.org/10.1145/3290607.3312879
Shenton, A.K. (2004). Strategies for ensuring trustworthiness in qualitative research projects. Education for Information, 22, 63–75. doi:10.3233/ EFI-2004-22201
Cite This Article
Delve, Ho, L., & Limpaecher, A. (2023c, April 6). What Is Consensus Coding and Split Coding in Qualitative Research? https://delvetool.com/blog/consensus-coding-split-coding